The Spread of the Code-Red Worm (CRv2)
An analysis by David Moore and Colleen Shannon on the spread of the Code-Red (CRv2) Worm. For more information contact info@caida.org
We would like to thank Pat Wilson and Brian Kantor of UCSD for data and discussion; Vern Paxson (LBL and ACIRI) for providing an additional view point of data; Jeff Brown (UCSD/CSE) for producing animations of worm spread; Ken Keys (CAIDA) for development of graphs and discussion; Bill Fenner (AT&T Research) for useful comments and fli2gif; and Stefan Savage (UCSD) and kc claffy (CAIDA) for suggestions. Support for this work was provided by DARPA ITO NGI and NMS programs, NSF ANIR, and Caida members.
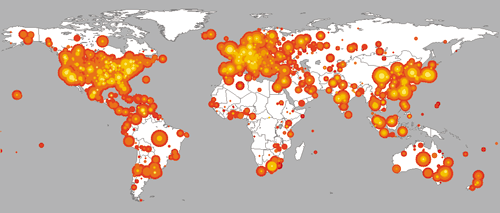
This quantitative illustration shows the early (yellow), middle (orange), and late (red) stages in the spread of the Code Red worm on July 19.
Overview
On July 19, 2001 more than 359,000 computers were infected with the Code-Red (CRv2) worm in less than 14 hours. At the peak of the infection frenzy, more than 2,000 new hosts were infected each minute. 43% of all infected hosts were in the United States, while 11% originated in Korea followed by 5% in China and 4% in Taiwan. The .NET Top Level Domain (TLD) accounted for 19% of all compromised machines, followed by .COM with 14% and .EDU with 2%. We also observed 136 (0.04%) .MIL and 213 (0.05%) .GOV hosts infected by the worm. An animation of the geographic expansion of the worm is available.
- UK mirror
Mirror Site for Animations
Animations created by Jeff Brown (UCSD CSE department),
based on analysis by David Moore (CAIDA at SDSC).
Copyright UC Regents 2001.
Background and Methodology
Around 10:00 UTC in the morning of July 19th, 2001 a random seed variant of the Code-Red worm (CRv2) began to infect hosts running unpatched versions of Microsoft's IIS webserver. The worm spreads by probing random IP addresses and infecting all hosts vulnerable to the IIS exploit. As noted by others, there are at least two variants of the worm: one that used a fixed, static seed for its random number generator, and another that used a random seed. [eeye] [stuart] In this paper, we examine the characteristics of the random seed variant of Code-Red (CRv2).
This analysis covers spread of the worm during the 24 hour period beginning July 19th at midnight UTC. The data used for this preliminary study were collected from two locations: a /8 network at UCSD and two /16 networks at Lawrence Berkeley Laboratory (LBL). Two types of data from the UCSD network are used to maximize coverage of the expansion of the worm. Between midnight and 16:30 UTC, a passive network monitor recorded headers of all packets destined for the /8 research network. After 16:30 UTC, a filter installed on a campus router to reduce congestion caused by the worm blocked all external traffic to this network. Because this filter was put into place upstream of the monitor, we were unable to capture IP packet headers after 16:30 UTC. However, a second UCSD data set consisting of sampled netflow output from the filtering router was available at the UCSD site throughout the 24 hour period. Vern Paxson provided probe information collected by Bro on the LBL networks between 10:00 UTC on July 19th and 7:00 on July 20th. Unless otherwise specified, we have merged these three sources into a single dataset to produce the following results.
Host Infection Rate
We detected over 359,000 unique infected hosts in this 24-hour period. Hosts were considered to be infected if they sent TCP SYN packets on port 80 to nonexistent hosts on these networks. To determine the rate of host infection, we recorded the time of the first attempts of each infected hosts to spread the worm. Because our data represent a sample of all probes sent by infected machines, the number of hosts monitored provides a lower bound on the number of hosts that have been compromised at any given point in time. Figure 1 shows the number of infected hosts over time as monitored on the UCSD and LBL networks. The growth curve of the hosts measured with passively tapped packet headers is steepest because the monitor sees all packets destined for approximately 1/256th of the address space. The curve based on the netflow data grows more slowly because the netflow data represents a sampling of all traffic, and some flow export packets were dropped before being recorded. The LBL curve increases more slowly because although Bro monitors all packets, it observes a much smaller portion of the address space. Assuming random selection of addresses probed by infected hosts, a larger address space will be probed more often than a smaller one. All of the horizontal (no growth) segments on the curves are caused by periods in which we were unable to collect data.
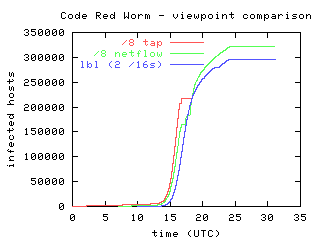
Figure 1
Figure 2 shows the number of infected hosts over time across all three data sources. The growth of the curve between 11:00 and 16:30 UTC is exponential, as can be seen in the logarithmic scale plot (Figure 3). On the surface, it seems to fit reasonably with the growth model for the worm infection proposed by Stuart Staniford. Discrepancies between the upper ranges of the growth model and our data are caused both by the fixed cutoff time of the worm itself and by hosts repaired or isolated throughout the day.
![]() Figure 2 |
![]() Figure 3 |
Figure 4 provides a more detailed view of the spread of the worm in terms of the number of new hosts seen in 1 minute periods throughout the day. Unfortunately, the peak of the initial curve occurs at about the same time that the passive monitor data became unavailable, so the duration of the 2,000 host/minute infection rate is unknown. In particular, the large spike of 7,700 hosts is an anomaly caused by a small gap in the collected netflow data that resulted in measurement of all hosts infected during the downtime at the time collection resumed. Thus the spike in the number of hosts infected is actually representative of all the hosts infected between 16:51 and 17:21 UTC. In actuality, we believe that the infection rate from 16:30-18:00 UTC tapered smoothly.
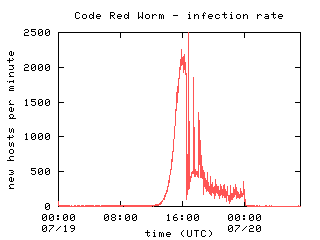
Figure 4
Again, 359,104 hosts were compromised in approximately 13 hours. Although the growth was slowing, had the worm not been programmed to stop spreading at midnight, additional hosts would have been compromised. The infection rate would have continued to decrease once the vast majority of vulnerable machines were infected. We speculate that the memory resident status of this worm would have allowed reinfection of many hosts.
Host Deactivation
During the course of the day, many initially infected machines were patched, rebooted, or filtered and consequently ceased to probe networks for vulnerable hosts. We consider a host that was previously infected to be inactive after we have observed no further unsolicited traffic from it. Figure 5 shows the total number of inactive hosts over time. The majority of hosts stopped probing in the last hour before midnight UTC on July 20th. At midnight, the worm was programmed to switch from an `infection phase' to an `attack phase', so the large rise in host inactivity is due to this design. The end of day phase change can be seen clearly in Figure 6, which shows the number of newly inactive hosts per minute. As in previous graphs, the spike near 16:30 is due to a gap in data collection.
![]() Figure 5 |
![]() Figure 6 |
Host Characterization
To understand the attributes of hosts compromised by Code-Red (other than the fact that they were running unpatched versions of IIS on Microsoft Windows platforms), we examined the domains, geographic locations, and top level domains (TLDs) of the infected hosts. Table 1 shows the breakdown of hosts by country, as placed by Ixia's IxMapping. Surprisingly, Korea is the second most prevalent source country of compromised machines, with 10.57% of all infected hosts.
Top 10 Countries Country # % -------------------------- US 157694 43.91 KR 37948 10.57 CN 18141 5.05 TW 15124 4.21 CA 12469 3.47 UK 11918 3.32 DE 11762 3.28 AU 8587 2.39 JP 8282 2.31 NL 7771 2.16 |
Table 2 provides a breakdown of infected machines by TLD. NET, COM, and EDU are all represented in proportions roughly equivalent to their share of all existing hosts. [netsizer] We also observed 136 MIL and 213 GOV hosts infected by the worm. Approximately 50% of all infected hosts had no reverse DNS records, so they were unable to be classified by the their domain names. These included, for example, the 390 hosts with addresses in 10.0.0.0/8. These machines were probably on private networks and were infected via either an external interface or another machine accessible via both internal and external networks. This suggests that many more hosts on internal networks may have been compromised.
Top 10 TLDs TLD # % -------------------------- Unknown 169584 47.22 net 67486 18.79 com 51740 14.41 edu 8495 2.37 tw 7150 1.99 jp 4770 1.33 ca 4003 1.11 it 3076 0.86 fr 2677 0.75 nl 2633 0.73 |
Table 3 shows the individual domains with the most infected hosts. We note that the top domain names are providers of home and small business connectivity, suggesting that hosts maintained by individuals at home are an important aspect of global Internet health.
Top 10 Domains Domain # % ------------------------------------ Unknown 169584 47.22 home.com 10610 2.95 rr.com 5862 1.63 t-dialin.net 5514 1.54 pacbell.net 3937 1.10 uu.net 3653 1.02 aol.com 3595 1.00 hinet.net 3491 0.97 net.tw 3401 0.95 edu.tw 2942 0.82 |
Animations
To help get a feel for the global impact of the worm and its growth, Jeff Brown created an animation of the geographic spread of the worm in five minute intervals between midnight UTC on July 19, 2001 and midnight UTC on July 20, 2001. For the animation, infected hosts were mapped to latitude and longitude values using Ixia's IxMapping, and aggregated by the number at each unique location. The radius of each circle is sized relative to the infected hosts mapped to the center of the circle using the formula 1+ln(total-infected-hosts). When smaller circles are obscured by larger circles, their totals are not combined with the larger circle; the smaller data points are hidden from view. Although we attempted to identify the geographic location of each host as accurately as possible, in many cases, the granularity of the location was limited to the country of origin. We plot these hosts at the center of their respective countries. Thus, the rapidly expanding central regions of most countries is an artifact of the localization method.
The animation is available in three formats: flipbook/flic (207kB), QuickTime (200k, QTv3 or newer), or as an animated gif (4.1 MB)
Animations created by Jeff Brown (UCSD CSE department),
based on analysis by David Moore (CAIDA at SDSC).
Copyright UC Regents 2001.
- UK mirror
Mirror Site for Animations
Flipbook animation of geographic spread of Code-Red worm (Preferred format 207k .fli)
Note: The recommended way to view the flipbook format is to use xanim on a Unix platform, or QuickTime Player 5 on Macintosh and Windows boxes. Use the "open URL" feature of a QuickTime player and paste in the URL.
Quicktime animation of growth by geographic breakdown (200K .mov {requires QuickTime v3 or newer} )
Animated gif of geographic spread of Code-Red worm (4.1 MB .gif)
Note: The animated gif does not display correctly in all browsers.
Conclusions
The primary observation to make about the Code-Red worm is the speed at which a malicious exploit of a ubiquitous software bug can incapacitate host machines. In particular, physical and geographical boundaries are meaningless in the face of a virulent attack. In less than 14 hours, 359,104 hosts were compromised. The global Internet community dodged a bullet with the Code-Red worm: little damage was actually inflicted in the attack. The worm did no significant damage to the machines it infected. It had a preset cutoff time. Although it attempted to launch a Denial of Service (DoS) attack against www1.whitehouse.gov, it orchestrated the attack against the IP address of the server, rather than the domain name, and actually checked to make sure that port 80 at the whitehouse.gov IP address was active before launching the denial of service phase of the attack. These features made it trivially easy to disable the Denial of Service (phase 2) portion of the attack. We cannot expect such weaknesses in the design of future attacks.
This assault also demonstrates that machines operated by home users or small businesses (hosts less likely to be maintained by a professional sysadmin) are integral to the robustness of the global Internet. As is the case with biologically active pathogens, vulnerable hosts can and do put everyone at risk, regardless of the significance of their role in the population.
The Code-Red worm is a wake-up call. This exploit demonstrates clearly the need to keep machines up-to-date with security developments. This exploit also underscores the need to back up critical systems; the worm could easily have corrupted data, reformatted hard drives, or caused other irreparable damage. Indeed, in the final analysis, we should all be uncomfortable with the extent to which luck, rather than proactive diligence, maintains the stability of the Internet infrastructure.
Code-Red also provides the Internet community a chance to test its response to a virulent security threat with minimal long-term damage. There was, however, some unexpected collateral damage to infrastructure: printers, routers, switches, dsl modems, and other devices with web interfaces crashed, rebooted or were otherwise damaged by the worm's probes. We should assess our response to the attack -- How quickly and reliably can we disseminate news about the threat? How quickly can infected hosts be located, isolated, and repaired? In the case of the Code-Red worm, even windowsupdate.microsoft.com was infected, and many hosts were re-infected during attempts to patch them.
Finally, we should all be concerned that it seems to take a global, catastrophic incident to motivate us to respond to a known threat. The exploit was discovered on June 18, 2001 and the first version of the Code-Red virus emerged on July 12th, 2001. The truly virulent strain of the worm began to spread on July 17th, a full 29 days after the initial discovery of the exploit and four days after the detection of the first (static seed) attack. In the future, we cannot afford to remain complacent in the face of such blatant warnings.
Follow-up Survey
CAIDA performed a follow-up survey of IP addresses which were identified as having been infected with the Code-Red worm on July 19th, 2001. A random subset of the 359,000 IP addresses originally infected are examined each day to see if they are still vulnerable to the same bug in IIS. Results from this survey are available in our Code-Red: a case study on the spread and victims of an Internet worm paper.
Acknowledgments
We would like to thank Pat Wilson and Brian Kantor of UCSD for data and discussion; Vern Paxson (LBL and ACIRI) for providing an additional view point of data; Jeff Brown (UCSD/CSE) for producing animations of worm spread; Ken Keys (CAIDA) for development of graphs and discussion; Bill Fenner (AT&T Research) for useful comments and fli2gif; and Stefan Savage (UCSD) and kc claffy (CAIDA) for suggestions. Support for this work was provided by DARPA ITO NGI and NMS programs, NSF ANIR, and Caida members.
Glossary
- IP address space
- the set of all possible IP addresses.
- worm
- a program that connects to other machines and replicates itself. worms have the potential to both damage infected machines and to interfere with networks and services due to congestion caused by the spread of the worm.
- packet header
- the data at the beginning of each IP packet containing the source and destination IP addresses, as well as information about the type of data contained in the packet.
- IP packet
- The fundamental unit of data transmission across a network. A chunk of data and control information headed from a source host to a destination host.
- passive monitoring
- study of network behavior without generating or otherwise interfering with traffic on the network.
- router
- a machine designed to direct packets from their source host to their destination.
- seed
- a starting point for a random number generator. a static seed causes a random number generator to output the same sequence of numbers each time the generator is invoked, although the numbers themselves are random in that they have no predictable relationship to each other. a random seed uses an unpredictable starting point, so it generates a random sequence of random numbers, rather than a predictable series of random numbers.